Obtain news and background information about sealing technology, get in touch with innovative products – subscribe to the free e-mail newsletter.
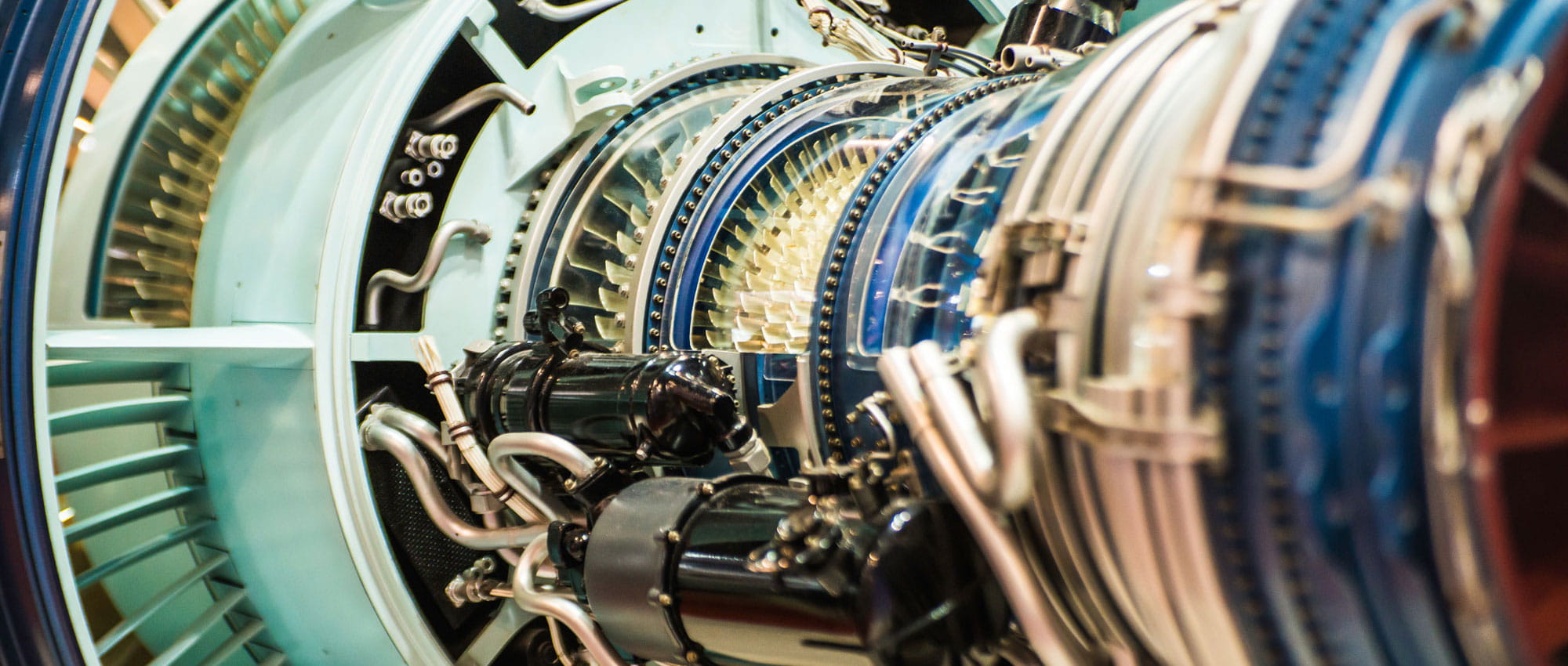
When the Drive Belt Calls the Technician
Predictive maintenance promises to bring an end to unforeseen machine breakdowns. Even if it is still a long way off, the developments are providing major benefits even today.
Maintenance was reactive in the beginning: First, a machine breaks down, then it’s repaired. The process was comparatively straightforward, but the breakdowns mostly came at inopportune times. Preventive maintenance emerged from this approach: Spare parts were swapped at regular intervals, based on experience. Estimates were made as to when components would fail. Fixed maintenance intervals provided reliability and the ability to plan ahead. But this was all theoretical. Since each machine is operated by different people in a different environment, the rates of wear will vary. Preventive maintenance either becomes too costly because it is carried out more frequently than it needs to be – or it doesn’t keep the machine from malfunctioning. Car owners certainly know a thing or two about this.
The Service Technician Is Already There
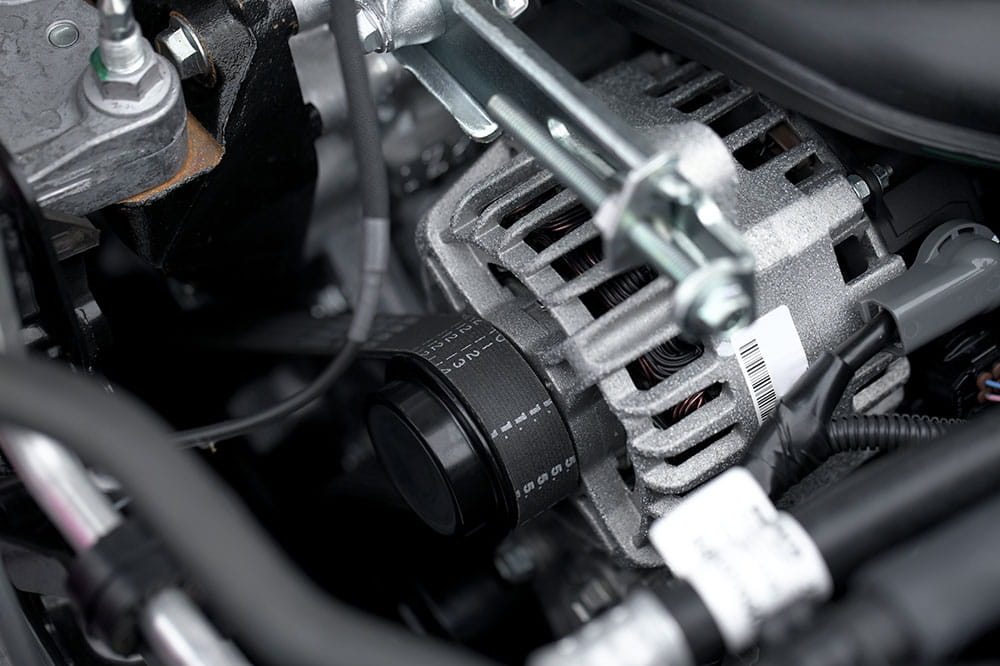
The holy grail for maintenance and repairs would be to service machinery just before something goes wrong – and not a moment later. The service technician would receive a notification on his smartphone in the evening, telling him that a drive belt would tear at 11:43 a.m. The sensors incorporated into the drive belts have been continually measuring the level of wear and sending data to a central computer on an ongoing basis. The technician is on site before the morning shift begins and replaces the part. In the era of Big Data, sensor technology and Industry 4.0, this would certainly be possible – or would it?
Even today, computers are collecting massive quantities of data. Sensors monitor individual components right on the machine, measuring temperature, moisture or vibrations, for example. With the right software, a determination of wear can be made using data reconciliation. On-time maintenance is an important cost factor: It reduces unplanned shutdowns, while cutting the cost of electricity and maintenance. Aircraft engines are equipped with sensors today – usually about 5,000 of them: 20 terabytes of data are collected every hour for evaluation. This pays off in industries like the aerospace sector where every hour of downtime costs an average of $10,000. The financial advantages of on-time maintenance quickly translate into millions of dollars in savings for a vehicle fleet. For some time, development engineers at Freudenberg Sealing Technologies have also been doing research on turning materials into sensors or even actuators, for example. They would be able to report their own level of wear – without impairing the seal’s original mission.
Improved Version of Old Concepts
At this point, these data evaluations aren’t just paying off for large machines. At Hannover Messe 2016, ABB was already presenting a solution for low-voltage motors. Predictive maintenance is again a key issue at the upcoming Hannover Messe 2019, which is devoting a special show to it, among other attractions. “Machine maintenance is well on the way to a new age,” the trade fair’s organizers say.
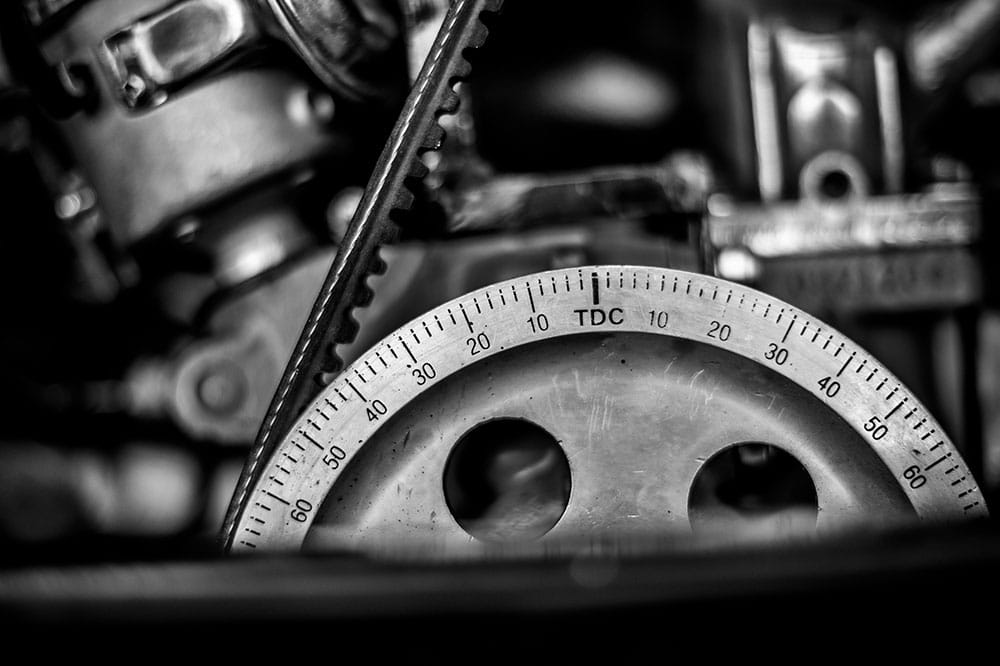
But depending on the definition, this type of maintenance still tends to be more preventive than actually predictive. More and more voices are questioning whether the original vision of predictive maintenance is actually achievable – or whether the result won’t inevitably be an improved, refined version of old concepts: remote maintenance and preventive maintenance. One argument is that a large share of the wear can be traced to operating errors. This makes the calculations more difficult. But there is also a counter-argument that the nonstop monitoring with sensors and computers leads users to better understand their equipment and vehicles. The wrong settings and operating errors can be read right from the data – and potentially changed. This yields an entirely new level of transparency with regard to their costs.
A key question remains: Where is the boundary between condition monitoring, that is, the monitoring of the level of wear, on one hand, and true predictive maintenance on the other? Is it predictive maintenance if the sensors promptly recognize that a belt has a tear on its surface? About 60 percent of German companies have had early experience with these approaches. The system is especially well-suited to machines that are installed as fixed equipment in one location and, as much as possible, are handled in the same way. If machines or vehicles are used in clearly different application areas or situations, their predictive power declines dramatically. For a while, the aviation industry has been discussing whether the right conclusions are actually being drawn from its massive amounts of data. It is clear that the mere collection of data is not enough. The crucial question is what algorithms are being used to interpret the information. And the right formula for each individual part has to be identified first.
Intelligent Data Instead of Data Mountains
The issue is anything but trivial. Engineers and data analysts must carefully assess whether, for example, the current collection of data is actually the right information and whether completely different sets of data and sensors might not be needed – and how a system becomes aware that bad data is in the process of being transmitted. The solution will involve structuring the data intelligently to derive useful answers from it. But if you want to increase the uptime of your equipment, you may not even need predictive maintenance to be your first step. It could be enough to monitor machine operation with the help of relatively small digitalization projects and draw your early conclusions from them. And there are already technical options for this kind of digital monitoring.
More Stories About Technology
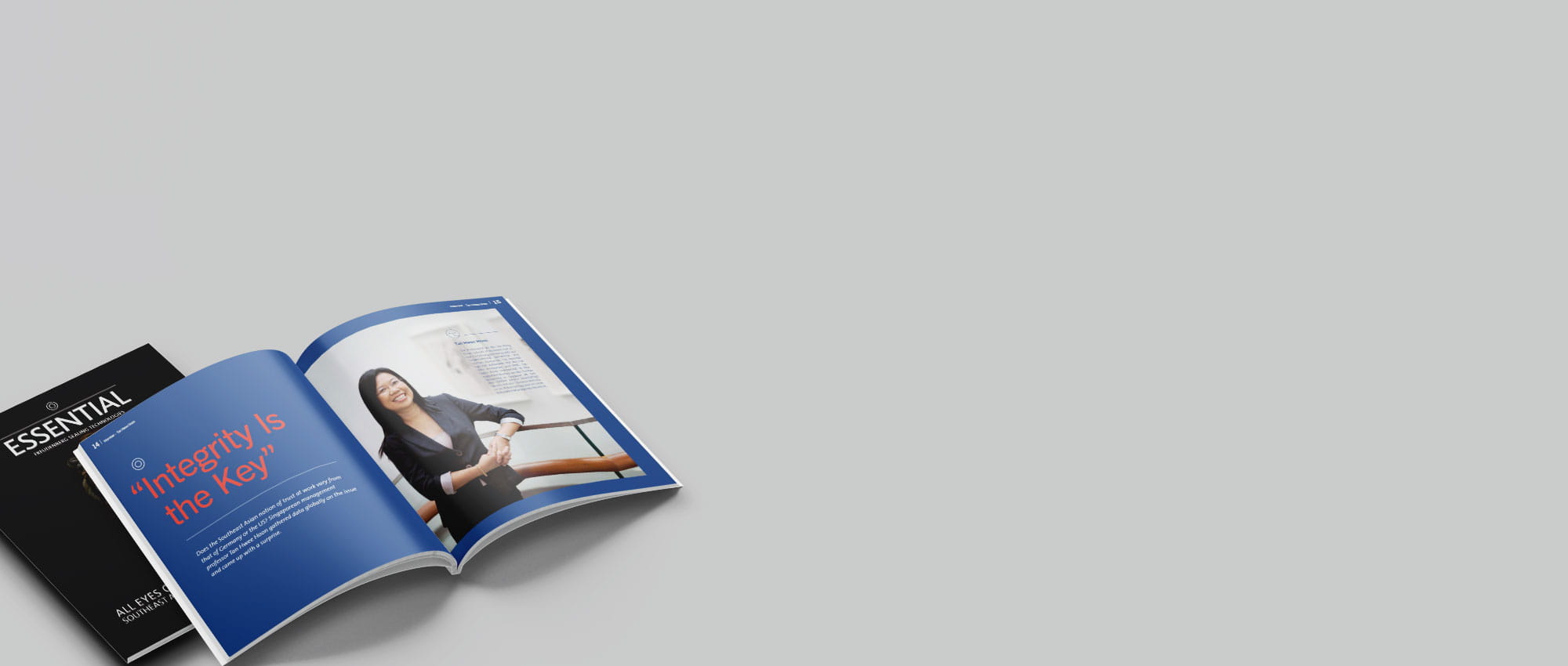